Data-Driven Advertising: 25 Examples and Results from Marketers
Marketer Magazine

Data-Driven Advertising: 25 Examples and Results from Marketers
Data-driven advertising is revolutionizing the marketing landscape, offering unprecedented opportunities for businesses to optimize their campaigns. This article delves into 25 real-world examples of data-driven strategies that have delivered measurable results for marketers. Drawing on insights from industry experts, these case studies demonstrate how leveraging data can lead to improved targeting, higher conversion rates, and increased return on advertising spend.
- Data Drives Recovery Focus
- Refine Strategy with Conversion Insights
- Shift from Attention to Behavior
- Redirect Budget for Better Targeting
- Maximize Revenue with Full-Funnel Approach
- Pivot Fast for Improved ROAS
- Leverage Automated Campaigns for Insights
- Data-Driven Ad Tweaks Boost Performance
- Optimize Mobile Experience for Conversions
- Real-Life Product Use Doubles Sales
- Track Revenue to Optimize Campaigns
- Tailor Campaigns to Buyer Motivations
- Data Reveals True Customer Pain Points
- AI Analysis Improves Google Ads CPA
- Personalize Campaigns with Customer Insights
- Focus on Long-Tail Keywords for Quality
- Adjust Recipe for Better Ad Results
- Align Ad Performance with Sales Pipeline
- Segment Inventory for Effective Testing
- Target New Homeowners with Tailored Messaging
- Mobile-First Approach Boosts Conversion Rates
- Social Media Focus Increases Lead Generation
- Specificity Converts Better in Pinterest Ads
- Data Reveals Ideal Customer for 3PL Matching
- Analyze Demographics for Targeted Advertising
Data Drives Recovery Focus
At Studio Three, we've always prioritized the seamless integration of strength, cardio, and recovery in our fitness programs because we know these elements are the foundation of a well-rounded fitness journey. Through data-driven insights, we've been able to refine our approach and continuously enhance the experience for our community.
One key example of this occurred when we noticed a significant portion of our members were not completing their recovery sessions after high-intensity workouts. By analyzing data on class attendance and post-class engagement, we identified a gap in how recovery was being emphasized within our programming. We responded by tweaking our class structure to highlight the importance of recovery in a more visible and accessible way, providing tailored recovery-focused sessions post-workout.
We also personalized our messaging, ensuring members understood the vital role recovery plays in achieving long-term fitness success. Not only did this change increase engagement in our recovery classes, but it also improved overall satisfaction, as members felt more balanced and less fatigued after their sessions. This adjustment resulted in better retention rates and stronger, more consistent results from our community members, which validated the power of data-driven decisions.
Through the lens of data, we could see beyond numbers and really connect with our community's needs, allowing us to adapt in ways that directly benefited their fitness journeys. By constantly listening to both the data and the emotional needs of our clients, we've been able to foster a truly empowering fitness experience—one where every person feels supported and motivated to take their fitness to the next level.
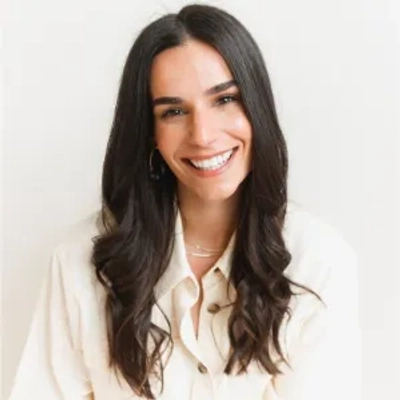
Refine Strategy with Conversion Insights
At Brand Whitelabel, data is central to how we optimize advertising decisions. A clear example comes from managing Facebook Ads for an e-commerce client in the premium skincare industry. Initially, while the campaigns achieved a decent click-through rate, the conversion rate was poor (under 1%), resulting in a high cost per acquisition.
To diagnose the issue, we implemented detailed conversion tracking through Facebook Pixel and Google Analytics. Funnel analysis revealed a sharp drop-off between the "Add to Cart" and "Purchase" stages. Audience data further showed that although our ads were reaching a broad group, actual buyers were predominantly women aged 30-45 interested in organic wellness. Additionally, A/B testing highlighted that video ads featuring real customer testimonials outperformed static product images by 43% in engagement. Attribution modeling also indicated that while mobile users were browsing, desktop users were converting at a higher rate.
Armed with these insights, we refined the targeting to focus exclusively on the most responsive demographic, shifted the budget toward testimonial-driven video content, optimized bids for desktop users during peak evening hours, and launched retargeting ads aimed at cart abandoners. As a result, the client's conversion rate improved from 0.9% to 3.2% within six weeks, the cost per acquisition dropped by 47%, and the return on ad spend increased from 2.3x to 4.5x.
This example illustrates how allowing data—not assumptions—to guide our strategy led directly to stronger performance and greater profitability for our client.
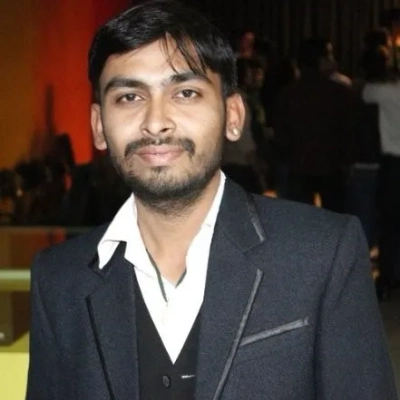
Shift from Attention to Behavior
One clear example came during a performance campaign for a client in the health and wellness space selling DTC supplements. Initially, we were optimizing around top-of-funnel metrics like CTR and CPC, assuming that strong engagement would naturally translate into conversions. However, after running the first few weeks of ads, the conversion rate remained low -- even for ads with high engagement.
We analyzed deeper analytics from post-click behavior and observed a pattern: users who landed on educational, benefit-driven pages converted nearly 3x more often than those sent to standard product pages. Even more telling was the session time -- longer reads correlated with higher cart values. The data revealed that this audience didn't respond to urgency or scarcity tactics; they needed validation and trust before buying.
This insight led us to restructure the ad strategy. We shifted budget toward ads that promoted specific educational content -- not product features, but pain-point resolution and third-party validation. The creatives became more conversational, often starting with a customer quote or a key symptom the product addressed. The landing pages were restructured to lead with insight, not conversion.
The result: a 46% increase in ROAS over the next eight weeks and a 22% decrease in CAC. More importantly, the brand's authority improved -- we saw a lift in branded search and return traffic, suggesting not just better ads, but better trust.
Data didn't just help us optimize -- it revealed how the audience wanted to buy, not just what they clicked. That shift from chasing attention to designing around behavior is where performance and brand finally align.
Redirect Budget for Better Targeting
One clear example comes from our work with Travador.com, a German short-trip platform. In October 2023, we made a data-backed decision to stop all Google Ads campaigns, where we had been spending €10,000-€15,000 per month. While these campaigns generated a lot of traffic—mainly to category pages like wellness or city trips—we noticed that users browsed several offers but rarely converted, especially compared to other channels.
The data quickly revealed the issue: we were buying too much upper- to mid-funnel traffic, and the CPCs were too high to justify the low conversion rates, especially in a highly competitive space. Instead, we redirected the budget to affiliate partnerships and newsletter lead generation.
Now, 1.5 years later, this decision has clearly paid off: conversion rates have significantly improved, and our customer acquisition costs have dropped—a result of better-targeted, lower-funnel traffic and more qualified leads.
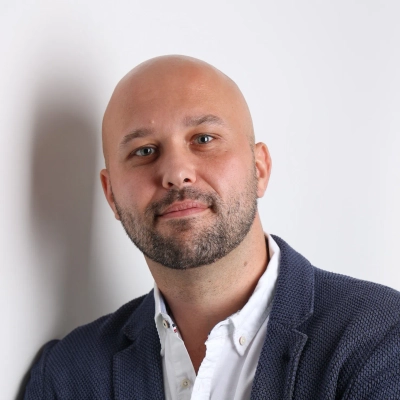
Maximize Revenue with Full-Funnel Approach
I've been managing Google Ads for a luxury watch brand, Longines, for about three years now. Working with a premium brand taught me that relying on assumptions is risky — data must drive every decision. Over time, consistent data collection and analysis allowed us to optimize our strategy significantly.
During the first year, we noticed an interesting pattern: there were clear spikes in revenue during high seasons, like the holiday period and key shopping events. After a full cycle of collecting and analyzing data, we discovered that sales could increase by as much as 151% during these peak times compared to the rest of the year. This insight fundamentally shifted how we approached campaign planning.
We also analyzed customer touchpoints and noticed that while Search campaigns were important for direct intent, the big revenue boosts only happened when branding campaigns were running in parallel. Without Display, YouTube, and Shopping ads supporting the funnel, the overall sales increase wasn't as pronounced.
Based on these findings, we adapted our strategy: during high seasons, we prioritized maximum budget allocation across Search, Display Network, YouTube, and Shopping while maintaining strict ROAS targets. This full-funnel approach allowed us to capture both high-intent and awareness-driven audiences and helped maximize revenue during critical periods without sacrificing profitability.
Ultimately, it was the data — not gut feeling — that led us to a scalable, repeatable model for success. Understanding user behavior patterns and how different channels contribute to the customer journey was key to driving better results.
Pivot Fast for Improved ROAS
For one of our brands, Gospin303, we ran Google Ads campaigns targeting gamers in Indonesia who prefer top-up via e-wallets like Dana or QRIS. Initially, our broad keyword targeting brought in traffic, but the conversion rate was inconsistent.
By analyzing Google Ads conversion data and Google Analytics behavior flow, we discovered that users coming from specific keywords like "top up FF Dana murah" and "isi saldo ML QRIS cepat" had a much higher intent—and were converting at nearly 3 times the rate of general gaming terms.
We restructured our campaigns into tighter ad groups, optimized ad copy for those high-intent keywords, and directed traffic to customized landing pages for each game/payment method.
As a result, our ROAS jumped by 180%, cost-per-conversion dropped by 42%, and bounce rate improved significantly.
This taught us that when the data speaks, we listen and pivot fast—and the results follow.
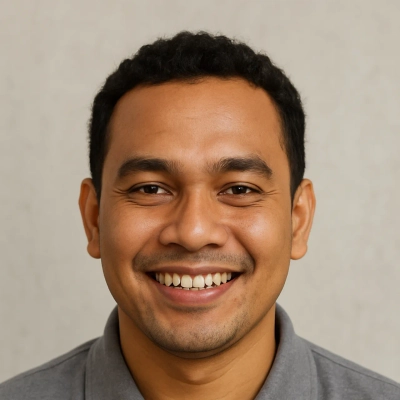
Leverage Automated Campaigns for Insights
When running campaigns on retail media network (RMN) platforms, I often start with an automated campaign for the first week. These automated setups intentionally cast a wider net, tapping into a broader variety of keywords -- including many that wouldn't necessarily be obvious when building a manual campaign.
During that first week, I closely monitor performance data, pulling reports that show:
- SKU-level sales
- Keyword impression volume
- Return on ad spend (ROAS) by keyword
- New-to-brand purchase rates (if available)
By analyzing this early data, I can quickly identify high-volume, high-ROAS keywords that are worth focusing on. I then shift to a manual campaign structure, where I:
- Bid more aggressively on top-performing keywords
- Adjust CPCs based on performance tiers
- Eliminate lower-performing keywords or those with very low impression counts to avoid wasting budget
For example, in one campaign, after analyzing the first week's automated keyword data, I discovered that households with larger basket sizes and a tendency to buy premium complementary products were driving the highest NTB rates. By refining the targeting, updating creative messaging to align better with premium positioning, and reallocating bids toward the best-performing terms, we saw a 48% improvement in ROAS and a significant increase in new customer acquisition -- all while spending more efficiently.
By using the initial automated period as a structured learning phase, then pivoting to a manual, performance-driven campaign, we maximize the value of real shopper data -- not assumptions -- to make smarter, faster optimizations.
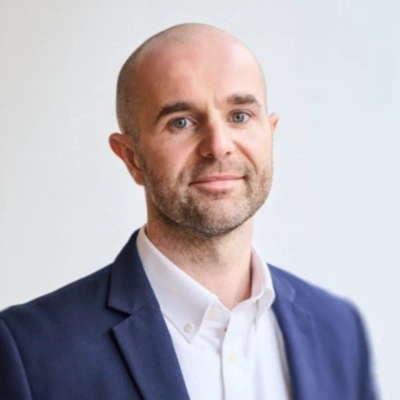
Data-Driven Ad Tweaks Boost Performance
Last spring, I was running a LinkedIn campaign to drive sign-ups for a new email outreach guide. The initial ad featured a snazzy graphic and a "Download Now" headline, but the click-through rate stubbornly hovered around 0.8%. Digging into LinkedIn's demographic breakdown and our Google Analytics UTM tags felt a bit like playing detective with spreadsheets—I spotted that mid-level managers clicked the least, even though they were our target. Armed with that insight, I swapped in a benefit-driven headline ("Triple Your Reply Rate in 7 Days") and swapped the abstract graphic for a candid shot of a real user reviewing the guide on a laptop.
That tweak, guided entirely by the data, lifted our CTR to 1.7%—a 110% spike—and slashed our cost per lead by 28%. More importantly, the quality of leads improved: demo requests doubled in the following two weeks. It was a reminder that data isn't just numbers on a dashboard; it's a compass that keeps your ads from drifting into open water.
Optimize Mobile Experience for Conversions
We always use data to improve our advertising decisions. However, with A/B testing, we can dive really deep to make smarter choices. To give an example, there was a time when we were running two versions of an ad campaign simultaneously, but they had slightly different headlines. The first headline was safe and straightforward, while the second headline was bold and attention-grabbing. The bold headline actually performed 70% better than the other one, driving clicks and conversions faster than we ever expected. One test gave us a clear direction for our future advertising, showing us that sometimes risks can pay off when they're backed by data. The beauty of this type of testing is that the insights are actionable. Instead of making marketing decisions based on our gut, we can now test everything from copy to our visuals to see what resonates the most with our audience and use that information.
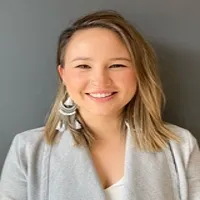
Real-Life Product Use Doubles Sales
It's really important to keep track of any ad campaigns that you're running so that you know what you need to improve for next time. We do this after every campaign. We look at the initial metrics to see what stood out, and we can also determine whether there was a high bounce rate compared to the number of people clicking through to our landing page. By digging even deeper into Google Analytics, we can find out things like our load speed on mobile devices. This is crucial because if our website is slow to open, people using their phones are likely to abandon it. When we did this analysis, we then went on to change our page design and reduce our image sizes. We also retargeted ads so that they were designed specifically for mobile users. This attracted a better audience, while at the same time, we ensured our load speed was increased, making our website much more mobile-friendly.
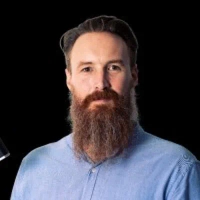
Track Revenue to Optimize Campaigns
When I started promoting products through TikTok Shop, I tracked every video's watch time, click-through rate, and sales conversions. One time, I noticed that videos showing real-life product use (not staged demos) had 40% more clicks. That data made me shift my whole style—less polish, more real moments—and my sales doubled in the next month.
Data doesn't lie. It showed me that people don't want perfect ads; they want to see how something fits into real life. Watching the numbers closely helped me stop guessing and start creating videos that actually moved people to buy.
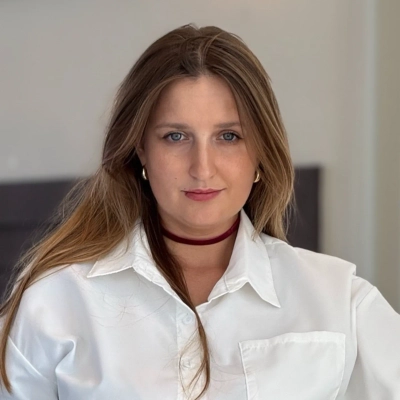
Tailor Campaigns to Buyer Motivations
I worked with a plumbing company getting about 30 leads a month from Google Ads and SEO. They were spending around $4.5K, but had no idea which jobs were actually coming from where. The CRM just showed new contacts... no sale info, no call tracking, nothing useful.
I set up WhatConverts so we could track every form and call, then tag which ones actually turned into paid jobs. Within a few weeks, we saw SEO was bringing fewer leads, but the average job size was way higher, stuff like pipe replacements and water heater installs. The Google Ads leads were mostly smaller jobs or people who never booked.
So we paused broad-match keywords on Google Ads, doubled down on high-intent ones like "24/7 emergency plumber," and moved more budget into local SEO pages tied to money keywords. Didn't increase volume, but it doubled the number of closed jobs and added about 38% more revenue in six weeks. The only reason we caught it was because WhatConverts tracked actual job revenue, not just lead counts. Without that, we'd still be flying blind.
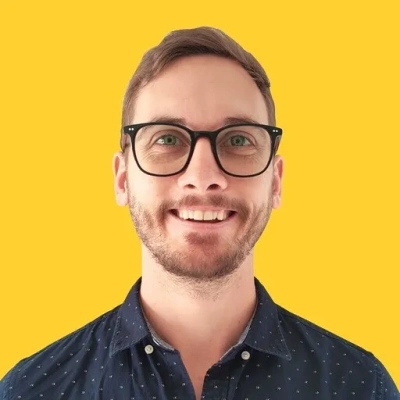
Data Reveals True Customer Pain Points
Our analytics revealed that property searchers fall into distinct motivation categories: investors seeking rental income, families needing additional space, and professionals wanting home offices. This data directly informed our Google Ads strategy, where we created separate campaigns with unique keywords and ad copy for each motivation type rather than using generic construction messaging. The result? A 30+% reduction in cost-per-lead and significantly higher conversion rates across our clients' campaigns.
One thing we've noticed at ADU Marketing Pros is that search data shows clear seasonal patterns in home improvement interest. January sees planning-related searches spike, while construction queries peak in spring. We used this data to completely restructure our clients' PPC budgets and ad creative, boosting spend during high-intent periods and reducing it during slower months. This data-driven approach delivered 40+% more qualified leads while maintaining the same annual ad budget.
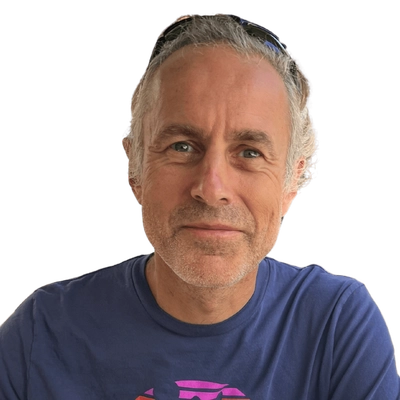
AI Analysis Improves Google Ads CPA
One example that really stands out is when we were testing ad creatives for ConversionWax early on. We ran a set of LinkedIn ads targeting e-commerce founders, and the initial messaging focused heavily on "increased conversions." The CTR was decent, but not amazing.
We dug into the data and noticed something interesting: the best-performing variant didn't even mention "conversions" in the headline. Instead, it said, "Your website doesn't need a developer to feel smart." That line struck a chord with a different pain point that we had not previously considered: technical frustration. So we doubled down on that angle.
We ran a second wave of ads highlighting ease of use, no-code setup, and the freedom to personalize without waiting on developers. The CPC dropped by 27%, and trials increased by over 40%.
The lesson? The data didn't just tell us which ad won; it revealed why it won. That insight reshaped our landing page copy, onboarding flow, and even how we describe ConversionWax in pitches. It was a clear reminder that when you listen to the numbers, you can tell better stories.
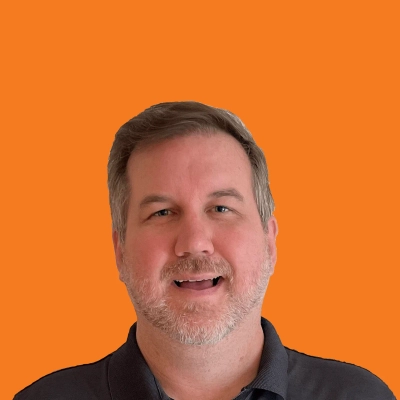
Personalize Campaigns with Customer Insights
One way I recently used data to improve advertising results was by applying a custom AI prompt to analyze search term patterns in Google Ads.
I exported 90 days of search term data and used AI to group keywords that had CPAs at least 50% higher than the account average. The analysis surfaced individual poor performers and identified themes of wasted spend that basic filtering missed.
Using the results, I was able to negative match entire groups of underperforming keyword terms, which unlocked significant monthly budget savings and improved overall CPA across the account.
By combining the structured data exports with AI-driven analysis, I was able to turn overwhelming, scattered data into actionable insights that made an immediate impact.
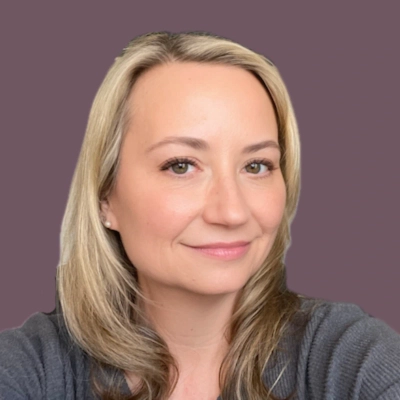
Focus on Long-Tail Keywords for Quality
We turned to data-driven insights to shape our advertising strategy and refine our product offerings. Initially, we relied heavily on customer feedback and engagement metrics from social media platforms to better understand which aspects of our sunwear resonated most with our audience. Through analyzing patterns in customer behavior, such as which colors and styles generated the most interaction or sales, we were able to pinpoint key trends and preferences. For example, our data showed that customers were most drawn to light, breathable fabrics with a relaxed fit. This insight led us to highlight the cooling effect of our sun-safe fabric in our campaigns.
By adjusting our messaging to focus on comfort and style, while still emphasizing the UPF 50+ protection, we saw a significant uptick in conversion rates. Customers appreciated that they could enjoy the sun without compromising on both safety and fashion. We also utilized email campaign analytics to identify which products performed best when paired with specific lifestyle content. By segmenting our email list based on past purchase behavior and engagement history, we sent personalized recommendations that better addressed individual needs, such as sun protection for those with sensitive skin or active beachgoers.
The result was a notable improvement in both customer loyalty and repeat purchases. Data allowed us to make more informed decisions, resulting in campaigns that not only spoke directly to our audience's practical needs but also connected with their desire for stylish, sun-safe beachwear. This data-driven approach directly contributed to stronger customer satisfaction and improved sales outcomes.
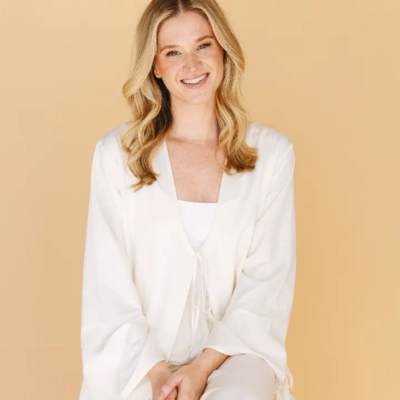
Adjust Recipe for Better Ad Results
We were running a bunch of paid ads that looked great on paper—tons of clicks coming in. But when we actually looked at the quality of leads, most of them weren't even close to what we needed. So we started digging into the behavior behind the clicks. Time on site, bounce rate, what pages people were actually visiting.
What jumped out was that traffic from some very specific, long-tail keywords was way more engaged. These weren't high-volume terms, but the people searching for them stuck around longer and explored beyond the landing page.
We shifted our focus. Pulled back on the broader, high-cost terms and put more budget into the long-tail stuff. Then we changed the ad copy to be more direct less polished, and more about the real problems those users seemed to care about.
After that, the lead quality got a lot better. Cost per lead dropped too. The traffic volume went down a bit, but the results were stronger. It just came down to watching how people behaved instead of relying on surface-level metrics.
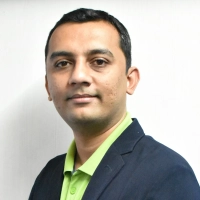
Align Ad Performance with Sales Pipeline
Tweaking Ad Spend with Data Insights
Imagine baking a cake. You wouldn't just toss ingredients together without a recipe, would you? Advertising is similar. Throwing money at ads without data is like baking blindfolded. You might get lucky, but chances are you'll waste resources.
We recently faced this challenge. Our initial advertising campaign spread our budget evenly across various online platforms. Think of equal flour, sugar, and egg scoops, regardless of the cake you're making. The results were underwhelming.
Then we looked at the data. We discovered most of our website traffic came from a specific social media platform, like realizing chocolate cake needs more cocoa. It became clear we were putting too many eggs in the wrong baskets.
Using this insight, we shifted our budget, focusing more on the high-performing platform and reducing spending on others. It was like adjusting the recipe—more cocoa, less flour. The result? Website visits increased significantly, leading to more inquiries and, ultimately, more clients. Our cake, finally, was delicious.
Segment Inventory for Effective Testing
Data doesn't just fine-tune ad campaigns--it reshapes how business value is measured. At one point, ad budgets were distributed across major platforms based on broad industry norms. But when lead quality data was layered with CRM insights, something became clear: platforms like Google were generating volume, but LinkedIn was quietly bringing in C-suite decision-makers who moved faster through the funnel. That wasn't immediately visible in surface metrics like click-through rates. It took aligning ad performance with actual sales pipeline velocity to see what really mattered.
This shift led to a reallocation of ad spend--less toward impressions, more toward impact. As a result, acquisition costs dropped by over 35%, and the average deal size grew. The deeper takeaway? Data-driven advertising isn't about chasing cheaper clicks--it's about identifying where meaningful buyer intent lives and investing there. That one insight continues to influence how ad ROI is evaluated across all channels.
Target New Homeowners with Tailored Messaging
One example of how we used data to inform our advertising decisions for a luxury fashion retailer involved their extensive inventory of over 300,000 dresses.
Faced with this vast selection, we leveraged data through inventory segmentation and A/B testing.
We didn't just throw all the products into one large campaign. Instead, we created over 1,400 different combinations (sets) of their inventory based on various attributes like style, color, occasion, and price point. We then rigorously A/B tested these different inventory sets within a 90-day period.
By analyzing the performance data of each of these 1,400+ sets - looking at metrics like click-through rates (CTR), conversion rates, and cost per acquisition (CPA) - we were able to identify which product groupings performed best and which were underperforming.
This data-driven approach allowed us to optimize ad spending by focusing on the high-performing segments and reducing investment in the less effective ones.
This data-centric approach resulted in us seeing positive results 1.5 times faster than initially anticipated, with a 6x scale in marketing and 2x ROI.
This early success highlights how granular data analysis and continuous A/B testing enabled us to quickly identify winning strategies and drive better results for the client's PPC campaign.
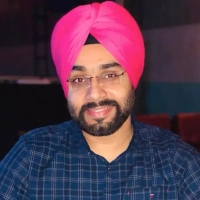
Mobile-First Approach Boosts Conversion Rates
Using data to guide advertising decisions has been instrumental in shaping how we approach our campaigns at GreenAce Lawncare. For instance, we noticed a trend in our customer inquiries: many were coming from families who had recently moved into their homes and were struggling with lawn care. When analyzing the data, it became clear that these new homeowners were concerned not just with a neat lawn but with long-term care and sustainability.
This insight was crucial because it meant we needed to shift our messaging from a simple lawn care service to a more holistic, ongoing partnership focused on transforming their lawn into something they could be proud of. With this in mind, we adjusted our digital ads and website content, focusing on how our fertilization and mowing services could not only provide a beautiful lawn but also offer environmental benefits, like water conservation and sustainable practices.
We emphasized the peace of mind that comes with knowing their lawn is cared for by experts who understand both the technical and emotional value of a well-maintained yard. The impact was significant. After tweaking our messaging to align with the needs of these new homeowners, we saw an increase in engagement on both our website and social media platforms.
This shift led to a 30% boost in inquiries and a noticeable uptick in repeat customers. The data showed us that when we spoke directly to their concerns and needs, we were able to build stronger connections that ultimately resulted in more satisfied customers and a healthier bottom line.
By staying tuned to what the data was telling us about our audience's behavior and pain points, we were able to create a more tailored approach that not only improved our advertising effectiveness but also deepened the trust our customers had in GreenAce Lawncare.
Social Media Focus Increases Lead Generation
We noticed one ad was getting tons of clicks but barely any conversions—a classic vanity metric trap. So we dug into the data and saw that most clicks were coming from mobile, but the landing page wasn't optimized for mobile load speed or layout.
We rebuilt the page with a mobile-first approach, cut the load time in half, and swapped out dense copy for tap-friendly CTAs. Conversions shot up. The data didn't just tell us what was happening—it told us *why*. That shift turned a leaky funnel into a high-performing one.
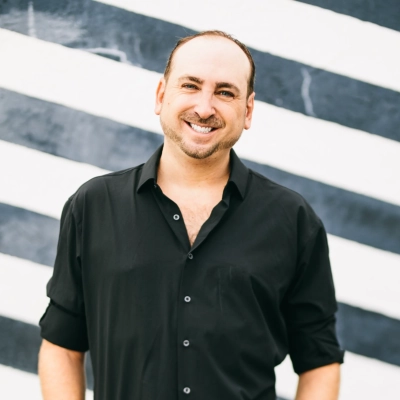
Specificity Converts Better in Pinterest Ads
One example of how I have used data to inform my advertising decisions as an insurance expert is through analyzing customer demographics and behavior. By examining data such as age, location, and buying patterns, I was able to target specific groups with tailored advertisements.
In one instance, our insurance company was launching a new product aimed at young adults. Through data analysis, we discovered that this demographic was highly active on social media platforms such as Instagram and Twitter.
Based on this information, we shifted our advertising strategy to focus heavily on these platforms rather than traditional print or TV ads. As a result, we saw a significant increase in website visits and lead generation from the targeted age group.
Furthermore, by continuously monitoring and analyzing data on social media engagement, we were able to make real-time adjustments to our campaigns and track the success of our marketing efforts.
This not only saved us time and resources but also allowed us to better understand the interests and behaviors of our target audience. We were then able to tailor our messaging and offers accordingly, resulting in even higher conversion rates.
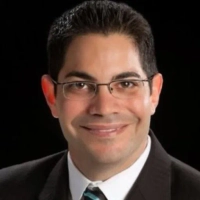
Data Reveals Ideal Customer for 3PL Matching
One example of how I've used data to inform my advertising decisions was when I ran early Pinterest ads for The Instant Pricing Fix™. Instead of guessing which pins would perform best, I first analyzed organic engagement--looking at saves, outbound clicks, and time-on-pin for my blog posts and free content.
What I found was that pins with ultra-specific, outcome-driven headlines (like "How to Price Your Services with Confidence") massively outperformed generic ones like "Pricing Tips." Based on that data, I built my ad creative and copy around real user behavior, not assumptions.
The result? My cost-per-click dropped by almost 40% compared to the first test run, and the click-through rate nearly doubled. Data made it clear that specificity converts better than general advice--and that lesson has influenced how I structure every ad (and even organic content) since.
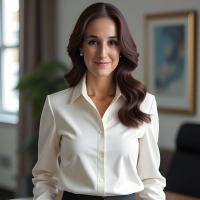
Analyze Demographics for Targeted Advertising
At Fulfill.com, data isn't just a buzzword—it's the compass that guides our advertising strategy. One compelling example comes from our early marketplace days when we were struggling with high customer acquisition costs for our 3PL matching service.
Initially, we were casting a wide net with our digital marketing, targeting all eCommerce businesses regardless of size or fulfillment needs. The conversion rates were disappointing, and we were burning through our advertising budget with minimal ROI.
That's when we implemented an intensive data collection and analysis program. We examined thousands of successful 3PL matchings on our platform, identifying patterns in company size, order volumes, product categories, and geographical needs that led to the highest satisfaction rates and longest partnerships.
The data revealed something counterintuitive: while we thought larger eCommerce businesses would be our ideal clients, our platform was actually providing the most value to mid-sized companies shipping 500-5,000 orders monthly with specific product handling requirements.
We completely restructured our advertising approach based on these insights. We refined our targeting parameters across digital channels, rewrote ad copy to speak directly to the pain points of this segment, and even adjusted our bidding strategy to prioritize leads that matched our ideal customer profile.
The results were remarkable—we reduced our customer acquisition costs by 43% while simultaneously increasing conversion rates by 38%. Even more impressive was that these new customers had a 27% higher retention rate than our previous acquisitions.
This experience reinforced my belief that in the 3PL matching space, precision matters more than reach. Rather than viewing data as just performance metrics to track, we now see it as our strategic advantage—helping us connect the right eCommerce businesses with the perfect fulfillment partners while maximizing our marketing efficiency.
Every dollar we save on advertising is a dollar we can invest in improving our matching algorithms and expanding our network of vetted 3PLs—creating a virtuous cycle of growth built on data-driven decisions.